Harnessing Uncertainty in Domain Adaptation for MRI Prostate Lesion Segmentation
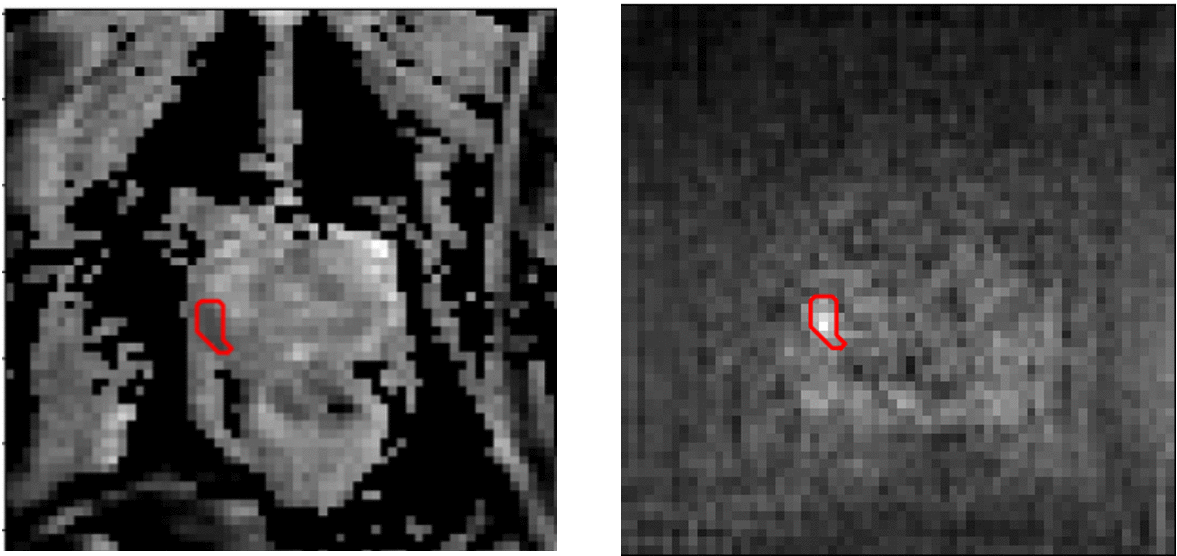
Abstract
The need for training data can impede the adoption of novel imaging modalities for learning-based medical image analysis. Domain adaptation methods partially mitigate this problem by translating training data from a related source domain to a novel target domain, but typically assume that a one-to-one translation is possible. Our work addresses the challenge of adapting to a more informative target domain where multiple target samples can emerge from a single source sample. In particular we consider translating from mp-MRI to VERDICT, a richer MRI modality involving an optimized acquisition protocol for cancer characterization. We explicitly account for the inherent uncertainty of this mapping and exploit it to generate multiple outputs conditioned on a single input. Our results show that this allows us to extract systematically better image representations for the target domain, when used in tandem with both simple, CycleGAN-based baselines, as well as more powerful approaches that integrate discriminative segmentation losses and/or residual adapters. When compared to its deterministic counterparts, our approach yields substantial improvements across a broad range of dataset sizes, increasingly strong baselines, and evaluation measures.
Method
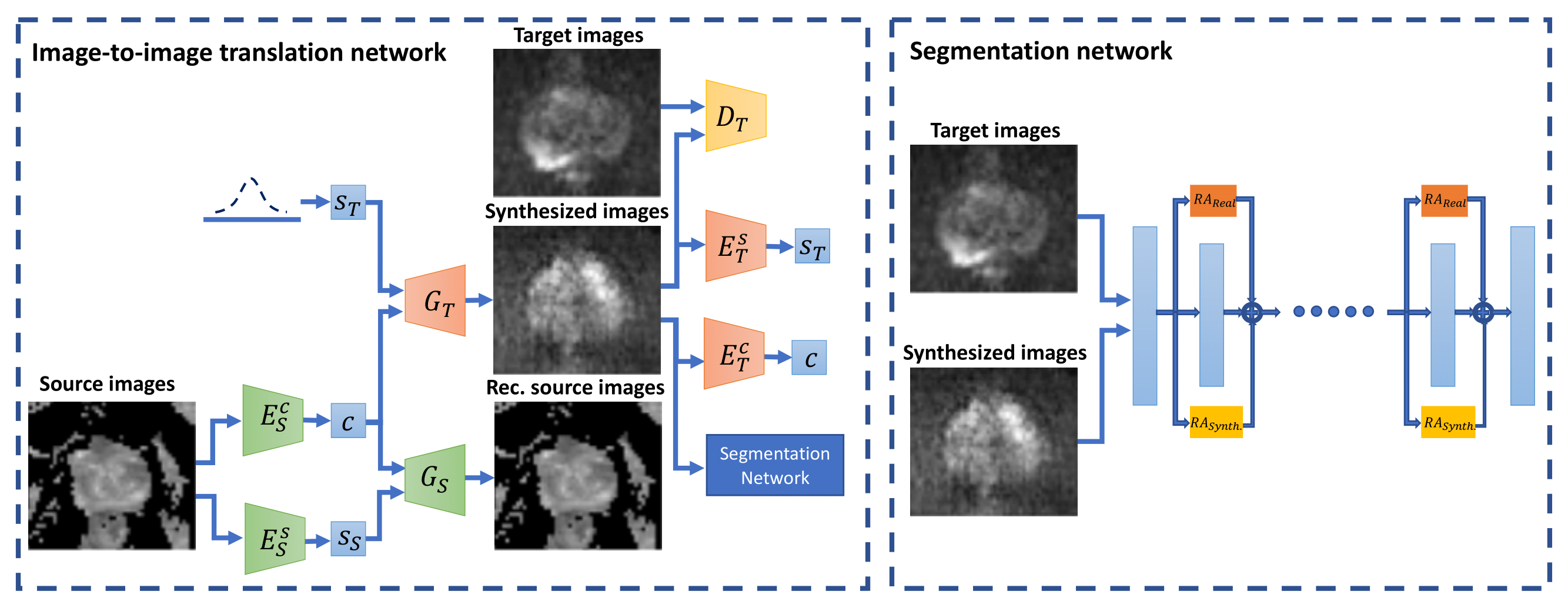
Results
Image-to-image transaltion results (Source->Target)
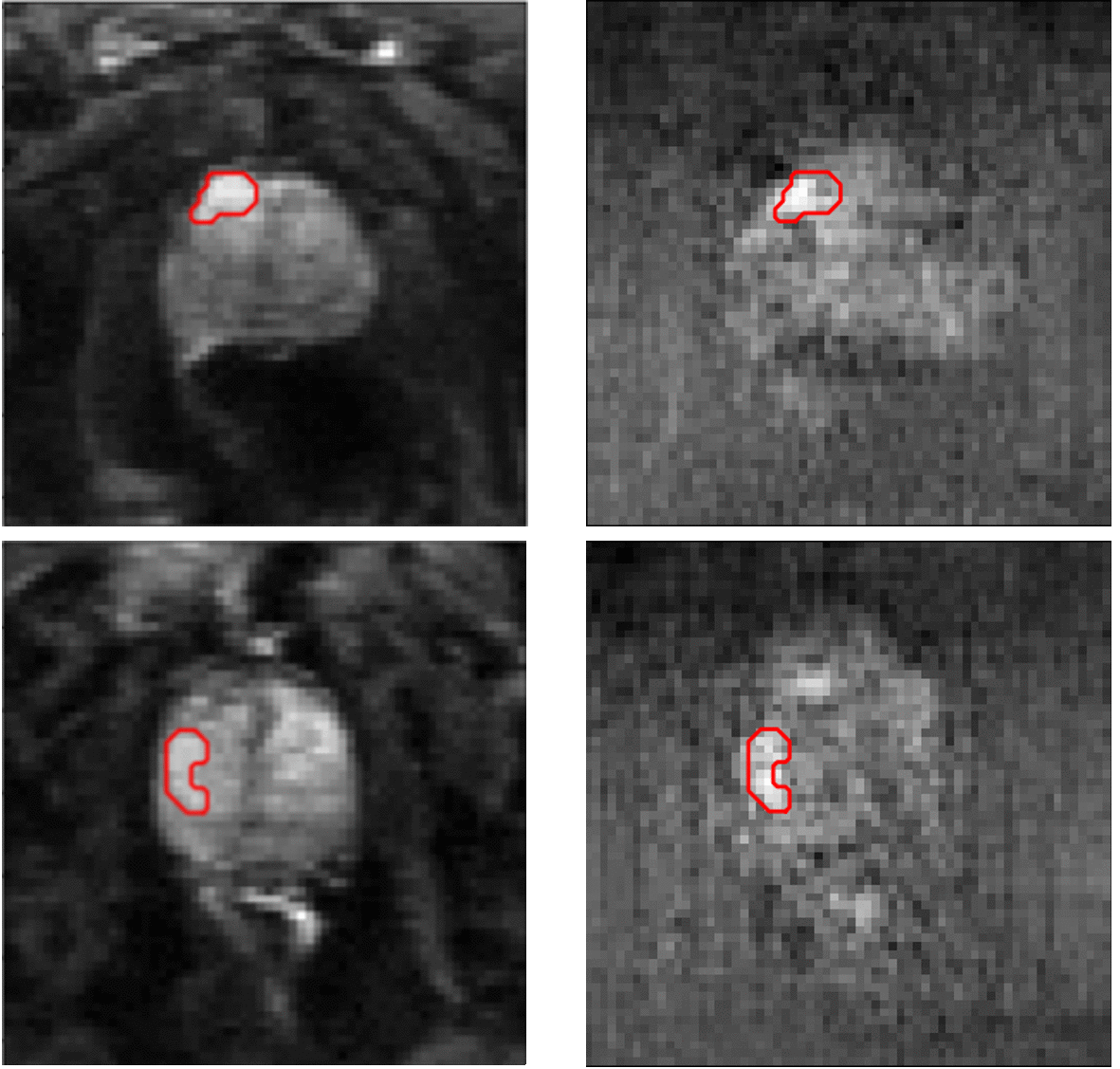
Segmentation results
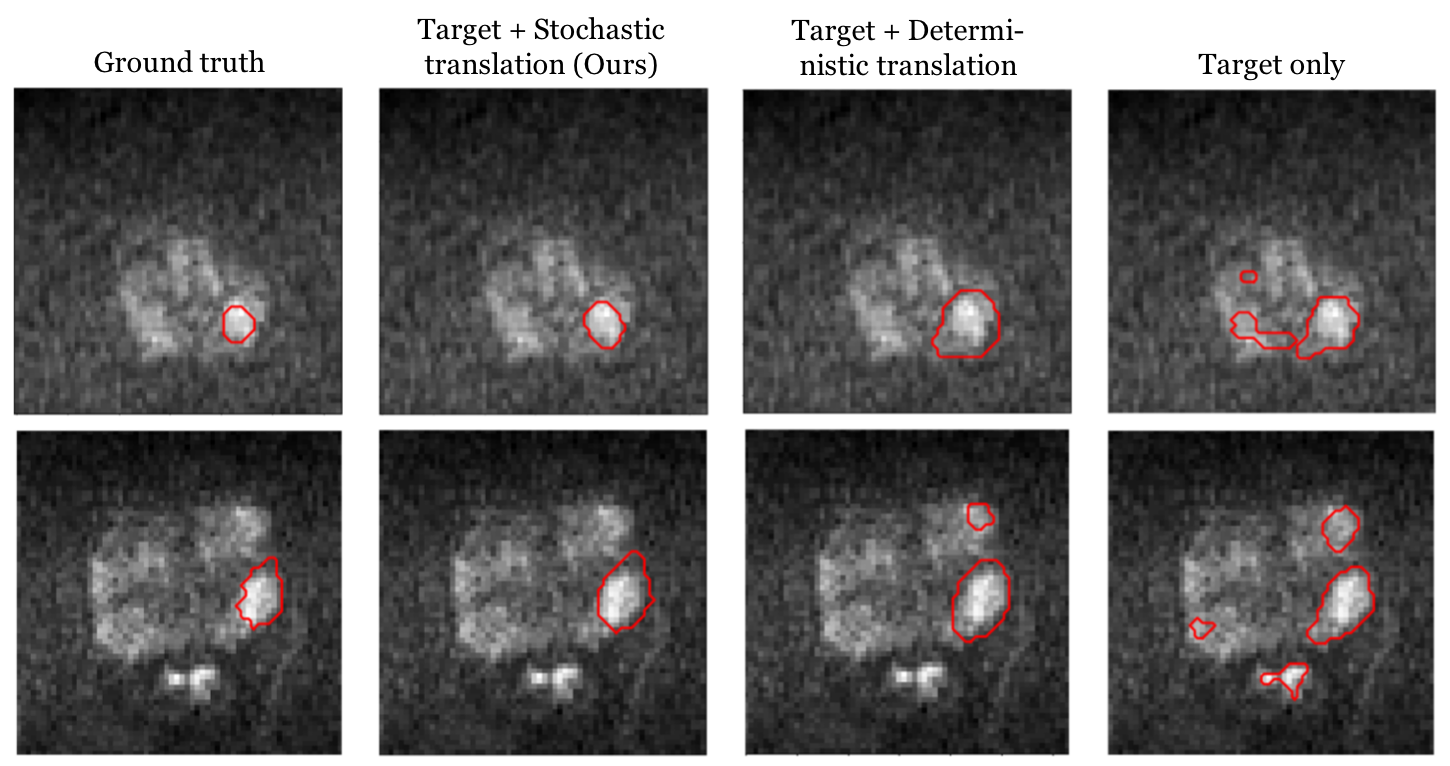
Material
Citation
@inproceedings{Chiou_20_harn_unc, title={Harnessing Uncertainty in Domain Adaptation for MRI Prostate Lesion Segmentation}, author={Chiou, Eleni and Giganti, Francesco and Punwani, Shonit and Kokkinos, Iasonas and Panagiotaki, Eleftheria}, year={2020}, booktitle={MICCAI}}